Few shot classification with Prototypical Networks
N shot classification is a task where the classifier has access to only N examples of each class in the test set. Solutions to this task are really useful in real world scenarios where a lot of labelled data is not present or new classes are being added frequently.
Prototypical Networks are a relatively simple method to perform this task, and they produce excellent results. They do so by mapping each data point to a representation vector. The vectors corresponding the N exmaples of each class are merged to create a prototype vector for each class. A test data point can be classified by computing its distances to prototype representations of each class.
Following is my re-implementation of the network in Pytorch.
References:
BaseModel
is a class containing a generic training loop and some functions that can be overwritten to execute any code at any point in the loop.
import tensorflow as tf # For keras like progress bars in the training loop :)
class BaseModel:
def __init__(self, ckpt_name='model.pt'):
self.ckpt_name = ckpt_name
self.start_epoch = 0
self.best_loss = 10000
def on_val_end(self):
val_loss = self.val_dict[self.monitor_metric]
if val_loss < self.best_loss:
self.best_loss = val_loss
self.save()
def save(self):
raise NotImplementedError
def on_train_start(self):
pass
def on_epoch_end(self):
pass
def on_fit_end(self):
pass
def on_epoch_start(self):
pass
def fit(self, dl, valid_dl=None, monitor_metric='val_loss', n_epochs=1):
self.dl = dl
self.valid_dl = valid_dl
self.n_epochs = n_epochs
self.monitor_metric = monitor_metric
self.on_train_start()
for epoch in range(n_epochs):
self.on_epoch_start()
self.epoch = epoch
self.n_batches = len(dl)
print(f'Epoch {epoch+1}/{n_epochs}')
pbar = tf.keras.utils.Progbar(target=self.n_batches)
for idx, batch in enumerate(dl):
self.batch_idx = idx
loss_dict = self.train_step(epoch, idx, batch)
pbar.update(idx, values=list(loss_dict.items()))
if valid_dl:
self.validate()
pbar.update(self.n_batches, values=list(self.val_dict.items()))
self.on_val_end()
else:
pbar.update(self.n_batches, values=None)
self.on_epoch_end()
self.on_fit_end()
Prepare datasets
ProtoData
is a class that creates batches for training and validation.
It needs to be initialised with a regular classification style pytorch dataset where each item is a tuple of (inputs, label).
It is very similar to a pytorch Dataset class except that the __getitem__
function returns a batch and not a single example.
To make it enumerable, the __getitem__
function raises an IndexError on index >= max length.
Algorithm for creating a single batch:
Given,
number of classes : nway
set of all labels : labels
number of support examples per class : nshot
number of query inputs per class : nquery
- select
nway
classes from the set of all labels - select
nshot
support inputs from each class - select
nquery
query inputs from each class - create a batch with the following order.
[class 1 support 1, class 1 support 2 ... class 2 support 1, class 2 support 2, ... class 1 query 1, class 1 query 2, ... class 2 query 1, class 2 query 2 ... ]
import torch
import random
class ProtoData:
def __init__(self, ds, nshot, nquery, nway, labels, num_batches):
self.nway = nway
self.nshot = nshot
self.nquery = nquery
self.labels = labels
self.num_batches = num_batches
self.ds = ds
self.d1 = ds[0][0].shape[0]
self.d2 = ds[0][0].shape[1]
self.d3 = ds[0][0].shape[2]
label_idx = {}
for i in range(len(ds)):
l = ds[i][1]
if l not in label_idx:
label_idx[l] = []
label_idx[l].append(i)
self.label_idx = label_idx
def __len__(self):
return self.num_batches
def __getitem__(self, idx):
"""
batch = [
class1_1, class1_2, ... , class2_1, class2_2, ... # supports
class1_query1, class1_query2,...,class2_query1, class1_query2,...]
"""
select_labels = random.sample(self.labels, self.nway)
if idx >= self.num_batches:
raise IndexError
bs = self.nway * self.nshot + self.nquery * self.nway
batch = torch.zeros(bs,self.d1, self.d2, self.d3)
for class_idx,c in enumerate(select_labels):
shuffled_idx = random.sample(self.label_idx[c], len(self.label_idx[c]))
selection = shuffled_idx[:self.nshot + self.nquery]
for selection_idx,i in enumerate(selection):
if selection_idx < self.nshot:
batch[class_idx*self.nshot+selection_idx,:,:,:] = self.ds[i][0]
else:
batch[self.nshot*self.nway + class_idx*self.nquery + (selection_idx - self.nshot),:,:,:] = self.ds[i][0]
return batch
Omnigot is a dataset used to benchmark few shot image classification methods. It contains single channel images of various characters from different languages.
import torchvision
ds = torchvision.datasets.Omniglot(
root='.', download=True, transform=torchvision.transforms.ToTensor()
)
from matplotlib.pyplot import imshow
import matplotlib.pyplot as plt
def tensor_to_img(x):
data = x.squeeze().numpy()
plt.figure()
plt.imshow(data, cmap='gray', vmin=0, vmax=1)
tensor_to_img(ds[900][0]) # plot single image
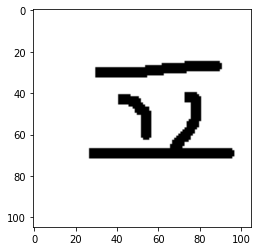
Create a prototypical network
The ProtoNet
class contains the entire training and validation algorithm.
It inherits from the BaseModel
class defined above, and overwrites the following functions.
- on_train_start : putting everything on GPUs, if available.
- train_step: processing a single batch of data and updating the encoder weights
- save and load : for saving and loading the state of the encoder and the optimizer
- on_epoch_end : running validation on unseen labels using 'few' labels
and printing the classification accuracy
The function train_step
executes the following steps.
- Split the batch into supports and queries. For N shot classification, we will have N support examples per class. The encoder network converts every support and query input into a representation vector.
- Calculate the mean of support representations from each class to create its prototype.
- Select the predicted class for a query input by picking the class with the nearest prototype to its representation.
- Calculate cross entropy loss with query example labels and logits. The logits for a query are negative distances of query representation from each prototype representation.
import numpy as np
import torch
import torch.nn as nn
import torch.nn.functional as F
class ProtoNet(BaseModel):
def __init__(self, enc, nshot, nway, nquery, lr=0.001):
super().__init__()
self.enc = enc
self.nshot = nshot
self.nway = nway
self.nquery = nquery
self.opt = torch.optim.Adam(self.enc.parameters(), lr=lr)
self.loss_fn = torch.nn.NLLLoss()
def on_train_start(self):
self.device = 'cuda' if torch.cuda.is_available() else 'cpu'
self.loss_fn.to(self.device)
self.enc = nn.DataParallel(self.enc).to(self.device)
self.epoch = -1
def compute_prototypes(self, support, k, n):
class_prototypes = support.reshape(k, n, -1).mean(dim=1)
return class_prototypes
def pairwise_distances(self, x, y):
""" Calculate l2 distance between each element of x and y.
Cosine similarity can also be used
"""
n_x = x.shape[0]
n_y = y.shape[0]
distances = (
x.unsqueeze(1).expand(n_x, n_y, -1) -
y.unsqueeze(0).expand(n_x, n_y, -1)
).pow(2).sum(dim=2)
return distances
def train_step(self, epoch, idx, x):
self.enc.train()
x = x.to(self.device)
embeddings = self.enc(x)
support = embeddings[:self.nshot*self.nway]
queries = embeddings[self.nshot*self.nway:]
prototypes = self.compute_prototypes(support, self.nway, self.nshot)
distances = self.pairwise_distances(queries, prototypes) # (num_queries, k_way)
# Calculate logits with softmax
log_p_y = (-distances).log_softmax(dim=1)
# labels = [class1 * nquery, class2 * nquery, ...]
y = []
for c in range(self.nway):
y.extend([c]*self.nquery)
y = torch.tensor(y).to(self.device)
loss = self.loss_fn(log_p_y, y)
self.opt.zero_grad()
loss.backward()
self.opt.step()
return {"loss": loss.item()}
def save(self):
torch.save(
{
"epoch": self.epoch+1+self.start_epoch,
"enc": self.enc.state_dict(),
"opt": self.opt.state_dict()
},
self.ckpt_name,
)
def load(self, ckpt_path):
ckpt = torch.load(ckpt_path)
self.opt.load_state_dict(ckpt['opt'])
self.enc.load_state_dict(ckpt['enc'])
self.start_epoch = ckpt['epoch']
def on_epoch_end(self):
if not hasattr(self, 'test_dl'):
print('No test dataloader')
return
nshot = self.test_nshot
nquery = self.test_nquery
nway = self.test_nway
self.enc.eval()
acc = []
bs = []
for x in self.test_dl:
x = x.to(self.device)
with torch.no_grad():
embeddings = self.enc(x)
support = embeddings[:nshot*nway]
queries = embeddings[nshot*nway:]
prototypes = self.compute_prototypes(support, nway, nshot)
distances = self.pairwise_distances(queries, prototypes, 'l2')
log_p_y = (-distances).log_softmax(dim=1)
y_pred = (-distances).softmax(dim=1)
preds = torch.argmax(y_pred, dim=1)
y = []
for c in range(nway):
y.extend([c]*nquery)
y = torch.tensor(y).to(self.device)
batch_acc = (preds==y).cpu().float().mean().item()
acc.append(batch_acc)
bs.append(x.shape[0])
numerator = sum([size * _ for size,_ in zip(bs,acc)])
denominator = sum(bs)
acc = numerator / denominator
print(f'epoch {self.epoch+1}: few shot accuracy {acc:.4f}')
Define a regular CNN as encoder. Create instances of training and validation datasets.
Experiment details (similar to the paper):
- 1 support example per class, for both training and evaluation
- 60 classes during training, 5 classes during evaluation.
- 5 query inputs, for both training and evaluation.
def conv_block(in_channels, out_channels):
bn = nn.BatchNorm2d(out_channels)
nn.init.uniform_(bn.weight)
return nn.Sequential(
nn.Conv2d(in_channels, out_channels, 3, padding=1),
bn,
nn.ReLU(),
nn.MaxPool2d(2)
)
class Convnet(nn.Module):
def __init__(self, x_dim=1, hid_dim=64, z_dim=64):
super().__init__()
self.encoder = nn.Sequential(
conv_block(x_dim, hid_dim),
conv_block(hid_dim, hid_dim),
conv_block(hid_dim, hid_dim),
conv_block(hid_dim, hid_dim),
conv_block(hid_dim, hid_dim),
conv_block(hid_dim, z_dim),
)
self.fc1 = nn.Linear(64,64)
self.fc2 = nn.Linear(64,32)
self.relu = nn.ReLU()
def forward(self, x):
x = self.encoder(x)
x = x.view(x.size(0), -1)
x = self.fc2(self.relu(self.fc1(x)))
return x
enc = Convnet()
nshot = 1
nway = 60
test_nway = 5
nquery = 5
model = ProtoNet(enc, nshot=nshot, nway=nway, nquery=nquery, lr=0.001)
proto_loader = ProtoData(
ds,
nshot=nshot,
nquery=nquery,
nway=nway,
labels=[i for i in range(900)], # labels 0-899 for training
num_batches=100
)
model.test_dl = ProtoData(
ds,
nshot=nshot,
nquery=nquery,
nway=test_nway,
labels=[(901 + i) for i in range(50)], # labels 901-950 for testing
num_batches=100
)
model.test_nshot = nshot
model.test_nway = test_nway
model.test_nquery = nquery
Train
model.fit(proto_loader, n_epochs=10)
Epoch 1/10
100/100 [==============================] - 35s 347ms/step - loss: 1.8391
epoch 1: few shot accuracy 0.8932
Epoch 2/10
100/100 [==============================] - 35s 348ms/step - loss: 0.7875
epoch 2: few shot accuracy 0.9136
Epoch 3/10
100/100 [==============================] - 35s 345ms/step - loss: 0.5544
epoch 3: few shot accuracy 0.9444
Epoch 4/10
100/100 [==============================] - 34s 345ms/step - loss: 0.4112
epoch 4: few shot accuracy 0.9524
Epoch 5/10
100/100 [==============================] - 34s 343ms/step - loss: 0.3292
epoch 5: few shot accuracy 0.9656
Epoch 6/10
100/100 [==============================] - 34s 344ms/step - loss: 0.2968
epoch 6: few shot accuracy 0.9608
Epoch 7/10
100/100 [==============================] - 35s 345ms/step - loss: 0.2524
epoch 7: few shot accuracy 0.9688
Epoch 8/10
100/100 [==============================] - 34s 345ms/step - loss: 0.2242
epoch 8: few shot accuracy 0.9648
Epoch 9/10
100/100 [==============================] - 34s 343ms/step - loss: 0.1958
epoch 9: few shot accuracy 0.9672
Epoch 10/10
100/100 [==============================] - 34s 343ms/step - loss: 0.2011
epoch 10: few shot accuracy 0.9664
Notes
- The omniglot dataset provided by torchvision and the one used by the authors of the original paper seem to be slightly different. The above code still performs well in 1 shot setup shown above and in my other experiments.
- If we expect the model to perform classification between
10 classes based on 2 examples/class, it is best to keep
nshot = 2
andnway >= 10
during training. - The distance metric between a prototype and a query representation can also be calculated using cosine distance, but the authors point out that euclidian distance works better.